Executive Summary
▪ This article is based on the final project for Professor Chen Haohan’s course “Data Science for Politics and Public Administration”. We trace the change of the public stance on the recent Israel-Palestine conflict from October 7 to 21, 2023.
▪ We use Information Tracer to gather popular tweets, R Studio to clean the datasets, OpenAI's API to identify the political stance of individual tweets (Pro-Israel, Pro-Palestine, and Neutral), and R Studio to visualize the results.
▪ Our research reveals a gradual shift in public sentiment towards a more Pro-Palestine stance during the aforementioned period. Our approach was influenced by Al-Agha and Abu-Dahrooj’s research on “Multi-level Analysis of Political Sentiments Using Twitter Data: A Case Study of the Palestinian-Israeli Conflict” (2019), wherein they attempted to determine the political stance of tweets, rather than their emotions (anger, sadness, fear, etc.).
Background
The recent escalation between Israel and Hamas in October 2023 has further heightened tensions rooted in the complex history of the region. The intensification unfolded when Hamas launched a barrage of rockets as well as a ground offensive into southern Israeli towns bordering the Gaza strip on October 7. Following which, Israel retaliated by enforcing a blockade on goods arriving to Gaza, as well as intense bombings and a ground offensive. The conflict has resulted in significant casualties on both sides and has had a devastating impact on civilians, particularly in Gaza, with a high number of fatalities and a substantial portion of the population displaced. The situation has sparked international concerns regarding the Israeli-Palestinian conflict, emphasizing the urgent need for diplomatic efforts to end the violence and find a lasting resolution.
Research Method
Data Gathering
We utilized the Information Tracer and downloaded the 500 most interacted with tweets related to specific inputted keywords for each day from October 7, 2023 to October 21, 2023. The keywords we used were “Israel”, “Palestine”, “Gaza”, “Hamas”, and “Conflict”. We specifically excluded instances of “Conflict” pertaining to Russia, Ukraine, China, and Taiwan, as we manually removed these words due to their frequent association with conflicts unrelated to the Israeli-Palestinian conflict. Our keyword selection aimed to minimize bias and encompassed the most commonly used terms associated with the Israeli-Palestinian conflict.
We considered other keywords such as “Terrorist”, “Middle East”, “Dead”, “Genocide”, “Biden”, and “Ceasefire”, but ultimately excluded them from the final dataset due to their potential bias towards a particular side, their broad nature, or the prevalence of neutral sentiments they resulted in.
Data Pre-processing
We conduct data analysis using datasets downloaded from Information Tracer in R Studio. Our process begins with data cleaning, where we select Twitter data and focus on the date and interaction count columns. We filter out non-English language tweets using the “textcat” package and clean up links, usernames, emojis, special symbols, and extra spaces in the tweets. Each tweet is also assigned a unique ID. After deleting duplicate tweets, the final dataset included a total of 3696 tweets which were split accordingly: “Conflict” (1126), “Gaza” (1120), “Hamas” (1192), “Israel” (1990) and “Palestine” (1037). Note that some tweets contained multiple keywords. Noticeably, “Israel” has almost double the number of tweets than “Palestine”, this is explained by the interchangeable usage of the words “Hamas” and “Palestine” in many tweets.
Labeling
Two researchers on the team manually labeled 150 tweets to Pro-Israel, Neutral and Pro-Palestine based on the following instructions:
Pro-Israel Tweets
- Tweets that express appreciation, praise, or support for Israel, the Israeli government, Benjamin Netanyahu, or the Israel Defense Forces (IDF).
- Examples include phrases like "I love Israel", "Israel has the right to defend itself", or any negative comments about Palestine, Hamas, or Palestinians.
- Look for Israeli naming conventions, e.g., "Palestinian terrorists", "Judea and Samaria", "IDF army".
Neutral Tweets
- Tweets that present a balanced view, factual information, or are unrelated to the conflict.
- Examples include neutral statements like “Both Hamas and Israel have committed war crimes”, “All lives matter, Israeli or Palestinian”, or factual updates like casualty numbers or unrelated news about Israel.
Pro-Palestine/Hamas Tweets
- Tweets that show support or sympathy for Gaza, Palestine, Hamas, or Palestinians.
- Examples include phrases like "Free Palestine", "It is called Palestine, not Israel", or any negative comments about Israel, the Israeli government, Benjamin Netanyahu, or the Israeli Army.
The set of instructions was fed into OpenAI’s developer portal for GPT-4 to systematically label the tweets. The similarity rate between GPT-4’s and human’s labeling is 83%. Note that many tweets failed to get labeled due to breaches to Microsoft Azure or OpenAI’s content guidelines.
Results and findings
Word Clouds
The word clouds below visualize the most common words found in tweets (excluding our key words), categorized by sentiment.
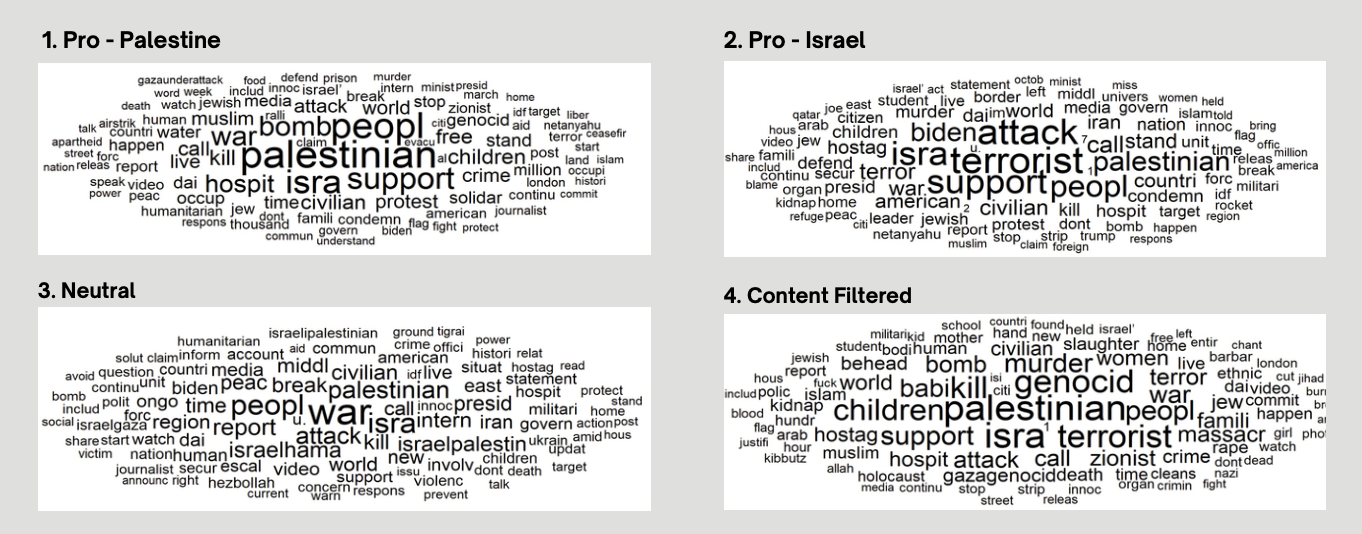
Pro-Palestine tweets prominently feature words such as “free,” “support,” and “hospital,” shedding light on the civilian situation in Palestine and emphasizing the humanitarian aspect of the conflict. On the other hand, Pro-Israel tweets tend to utilise words like “terrorist,” “attack,” and “Biden,” indicating a tendency to portray Israel's adversaries in a negative light and suggesting a connection between Pro-Israel sentiment and American politics. Neutral tweets, on the other hand, commonly employ words such as “war,” “report,” and “world,” suggesting a focus on finding solutions and potentially unbiased reporting of the conflict by global news outlets. As for tweets that were filtered and removed from the dataset, they contained prominent words like “genocide,” “terrorist,” and “massacre,” which were excluded due to their excessive violence or graphic nature.
Sentiment Distribution
The pie chart below provides a visual representation of the distribution of sentiments expressed in the tweets. It reveals that the largest portion of tweets, accounting for 37%, aligned with the Pro-Palestine sentiment. Following this, Neutral tweets constituted 28% of the dataset. In contrast, Pro-Israel tweets made up 19% of the total, which is approximately half the proportion of Pro-Palestine tweets. Finally, 15% of the dataset failed to get a labeling.
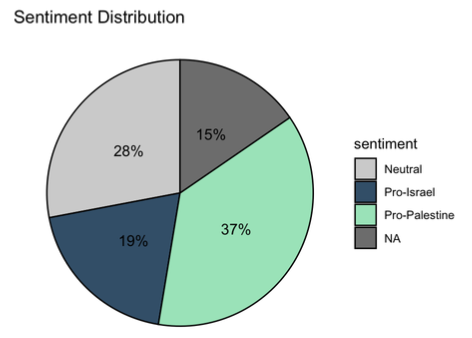
The stacked bar chart below provides a visual representation of the daily tweet activity categorized by sentiment. It reveals a notable trend of increasing Pro-Palestine sentiment from the 8th to the 21st of October, with the peak Pro-Palestine day occurring on the 14th of October. Conversely, the highest level of Pro-Israel sentiment was observed on the 7th of October, indicating a decline in sentiment as the time period progressed. Among the days analyzed, the 15th of October had the lowest level of interaction, while the 14th of October witnessed the highest level of engagement. It is worth mentioning that on the 14th of October, there were significant protests in Europe, Asia, and the US in support of Palestine and condemning Israel, as reported by Reuters in 2023.
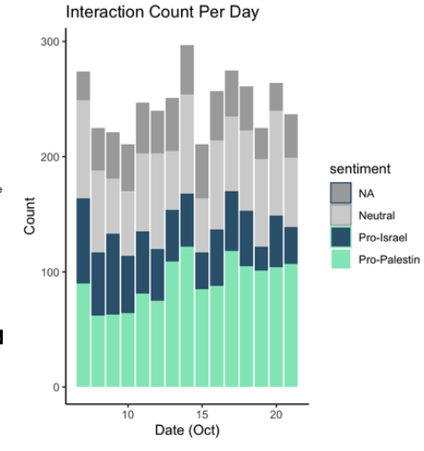
The scatter plot belwo illustrates the relationship between the interaction count and sentiment for each tweet. It reveals that Pro-Palestine tweets tend to receive higher levels of interaction compared to other sentiments, as indicated by the larger number of dots positioned above the 50,000 interaction mark. On the other hand, the graph demonstrates that Neutral tweets are less likely to garner interactions. This is likely due to the presence of numerous news articles among the neutral tweets, which may be less provocative in nature and generate fewer reactions. Additionally, neutral tweets often address the conflict in a balanced and unbiased manner, which could result in a lower level of engagement compared to more polarized sentiments.
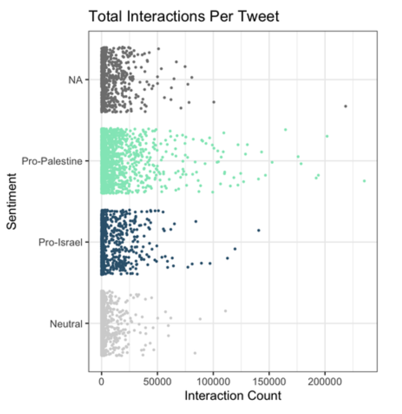
Example of most popular tweets
The three most interacted (total interaction count > 200,000) tweets are:
The German Chancellors entourage in Israel posted a viral video of them ducking under Hamas missiles prior to leaving an Israeli airport. Only problem? Their dramatic performance ACCIDENTALLY captured people in the background walking around as if nothing was happening.
We dont have photo evidence of 40 beheaded babies But we do have photo evidence of Israel targeting civilians in their utter leveling of Gaza.
IDFaffiliated accounts are sharing this video serve as proof that Hamas bombed the Gaza Baptist Hospital. Only problem? This video is from August of 2022.
Note that all three tweets contain graphic videos and photos, contributing to their virality. The phrase “beheaded babies” might be the reason why the second tweet triggered Azure OpenAI's content management policy and failed to get a labeling.
Sentiment Change Over Time
The combined time series graph below presents the changes in sentiment over the specified time period for each keyword. It reveals several noteworthy patterns.
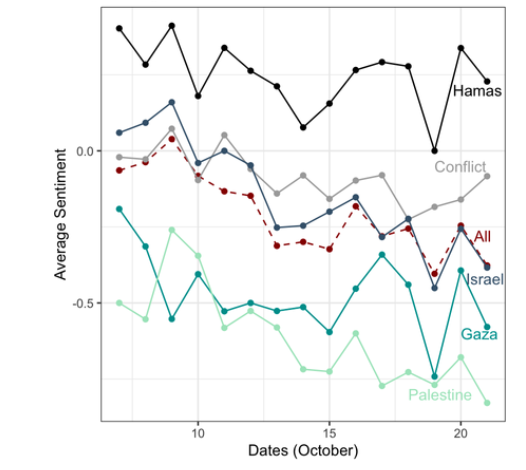
Firstly, among the keywords analysed, "Hamas" consistently maintained an averaged Pro-Israel sentiment, never dipping below a neutral stance (0). Secondly, "Palestine" emerged as the keyword with the highest Pro-Palestine sentiment. Conversely, "Conflict" displayed the most neutral sentiment throughout the time period.
On October 9th, the average sentiment for all keywords tilted towards Pro-Israel, marking a distinct shift. It is worth noting that the "Israel" line closely follows the "All" line, which can be attributed to the frequent use of the word "Israel" in tweets related to the conflict.
Additionally, the graph depicts a significant drop in Pro-Palestine sentiment on October 19th, potentially influenced by the tragic incident of Palestinian deaths resulting from a blast at al-Ahli Arab Hospital in Gaza, as reported by Al Jazeera in 2023.
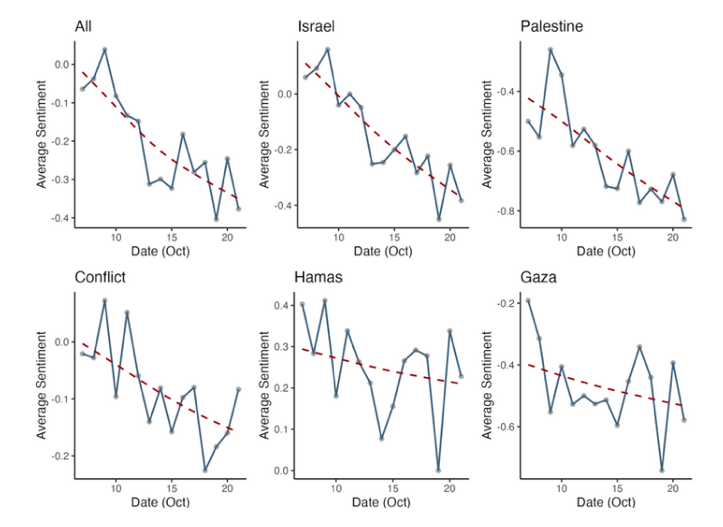
The individual time series graph above depicts distinct trends in sentiment for each keyword. The average trend line for "All" exhibits a noticeable shift towards Pro-Palestine sentiment over the course of the 15-day period. Similarly, the individual trend lines for each keyword also demonstrate a greater inclination towards Pro-Palestine sentiment.
Additionally, the graphs reveal that sentiment changes occurred at a faster rate for keywords such as "Israel," "Palestine," and "Gaza," as indicated by the Y-axis changing by 0.2 units instead of 0.1 units. On the other hand, "Conflict" emerges as the more neutral keyword, as reflected by the relatively low variation in sentiment, with a maximum difference of 0.3 units.
Final thoughts
Our analysis reveals a discernible shift in public sentiment towards the Pro-Palestine perspective over time. Nonetheless, it is important to acknowledge certain significant limitations in our study.
Firstly, our sentiment analysis was conducted solely on the most popular tweets, rather than encompassing the entire volume of tweets related to the issue. Consequently, the findings may not fully represent the actual sentiment of the public, but rather capture the prevailing discourse. Additionally, the limited number of keywords (five) used to gather tweets may not encompass a wide range of relevant opinions, potentially introducing bias towards a specific category. Furthermore, our chosen keywords may inadvertently include tweets unrelated to the Israel-Palestine conflict, resulting in their misclassification as "Neutral" and potentially skewing the overall percentage.
Secondly, in the process of data pre-processing, we removed links, images, and emojis, which could have influenced the sentiment conveyed in the tweets. Moreover, the use of the "textcat" package in R Studio to filter English language tweets may have introduced inaccuracies that we were unable to quantify.
Thirdly, the labeling of tweets may have been influenced by our own political stance and the instructions provided to GPT-4. Moreover, the accuracy of the results was impacted by Azure OpenAI's content management policy, which filtered out tweets containing extreme language, thereby potentially obscuring strong and clear stances on either side.
Finally, several professors have rightly pointed out that our research could be enhanced by considering the public sentiment and interaction count prior to October 7, 2023. This additional information may provide further insights into the impact of Hama's attack and contextualize the observed changes in sentiment uncovered in our research.
References
Al-Agha, I., and Abu-Dahrooj, O. (2019). Multi-level Analysis of Political Sentiments Using Twitter Data: A Case Study of the Palestinian-Israeli Conflict. Jordanian Journal of Computers and Information Technology (JJCIT), Vol. 05, No. 03.